We Prefer To Stay Grounded!
May 22nd 2024
Fuel costs in construction can account for up to 30% of the total project cost. Can embedded analytics help companies reduce these costs?
Can a holistic approach be used to identify ways to prevent carbon emissions?
GHG emissions in construction come from three sources: (a) direct emissions, (b) disturbances in vegetation, and (c) embodied carbon. We explore how a digital twin of the asset can provide a data-driven approach for optimized planning and operations.
We propose a three-pronged approach to identify sustainable practices during road construction:
Route Segmentation and Identification of Drive Phases: This includes the loading and dumping stages.
Use of Digital Elevation Models (DEM) and Drone Imagery (DTM): These tools measure the progress of construction through volumetric changes.
Use of Vegetation Indices Derived from Satellite Imagery: These indices calculate changes in carbon stock.
In this paper, we also discuss how these approaches can serve as the foundational elements in the creation of digital twins tailored to the construction industry.
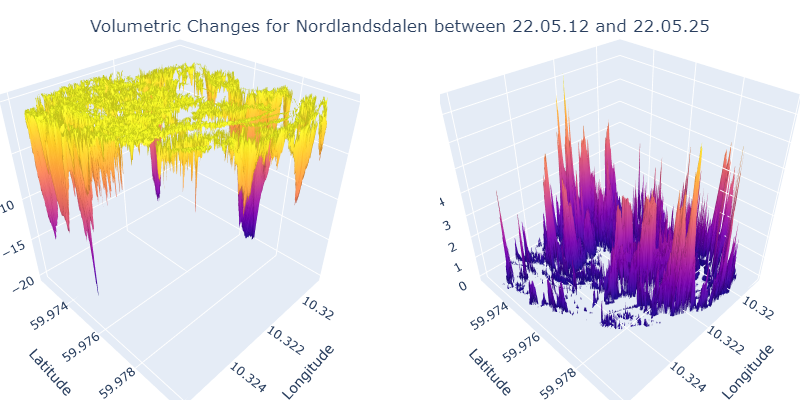
Route Segmentation and Driving Phase Identification
Providing drivers with real-time contextual feedback can considerably improve fuel efficiency. The low-footprint trip classifier developed by ThinkEvolve offers an easy-to-deploy system that can run on low-compute devices.
Contextual feedback involves providing drivers with real-time information based on their current driving conditions and behaviors. This information can include guidance on optimal speed, acceleration, braking, and gear shifting, tailored to the specific context such as road type, traffic conditions, and weather.
Automated Measurement of Construction Progress
We used drone imagery to capture the amount of mass moved during the construction phase. This was done using the Digital Elevation Model (DEM) from 2015 for the area of interest, which descends from the northeast to the southwest. To create a flat tunnel area with a buffer, trees at lower elevations were removed, and the ground was leveled using sand, stones, and dirt. Additionally, excavated material from the tunnel was temporarily stockpiled.
Using drone data, we first masked out the vegetation using a zero-shot ClipSeg model. Then, the DEMs were compared, and the volume of the mass dumped was computed. By correlating the dumping site with the quantity of mass dumped, we could measure progress on subsequent dates.
Use of Vegetation Indices to Measure Carbon Stock Change
We selected 2019 as the baseline year and compared it with estimates from 2022 to understand the changes in carbon sequestration potential. Due to the lack of suitable carbon stock estimation models for construction site areas, we relied on models from other geographies.
Next Steps
We have suggested low-compute techniques to reduce carbon footprint while preserving privacy. The trip-phase detector is designed to be lightweight and can provide alerts using limited computational power and bandwidth.
There is also a need to undertake on-the-ground surveys to tune the vegetation indices for the identify the density of the native tree species. Sampling is also required to approximate the density of the gravel for calculation of mass moved.
The three-pronged, data-driven approach offers a comprehensive method to assess the environmental impact of road construction. By utilizing diverse datasets, from GPS to drone imagery, we provide an alternative to deep learning approaches. This research emphasizes the importance of direct data insights for sustainable road construction. We also stress the need for creating ground truths and appropriate benchmarks for validating the results of our analysis.
Acknowledgements:
We thank Katarzyna Michalowska from SINTEF for her valuable feedback and input during the challenge. We sincerely thank all the members from NORA, SkanskaNorge AS, Ditio, SINTEF and all partners for their constant support throughout the challenge.
Publication URL: https://journals.uio.no/NMI/article/view/10720
DOI: https://doi.org/10.5617/nmi.10720